Listen to this article
|
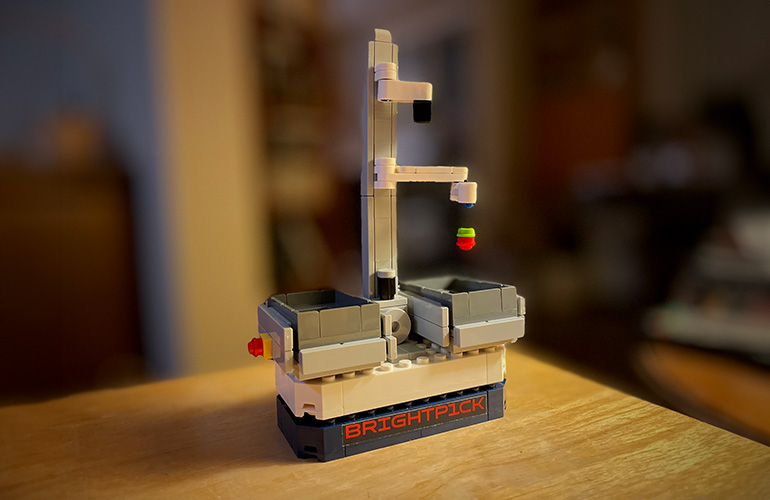
Brightpick applies machine vision and AI to robotic picking. Credit: Mike Oitzman
The Robot Report Podcast recently featured a conversation with Photoneo’s Jan Zizka about the new BrightPick fulfillment solution that the company released earlier this year. Below is an edited transcript of the conversation.
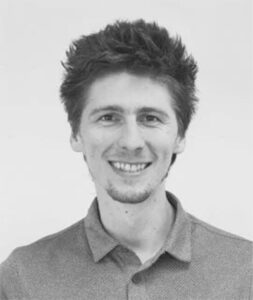
Jan Zizka, co-founder and CEO of Brightpick. | Credit: Brightpick
Zizka is the co-founder and CEO of Brightpick and its parent company, Photoneo Brightpick Group. He has filed more than 20 patents, ranging from 3D sensing methods to mobile robotics. Zizka is also a recognized expert in the fields of artificial intelligence, machine vision, and warehouse automation.
Photoneo’s customers include General Motors, Volkswagen, and KUKA. Before co-founding that company, Zizka was a research engineer at Micro-Epsilon, a leading sensor manufacturer.
He earned a Ph.D. in computer vision from Comenius University, during which he also did research on computational cameras and optics at the Massachusetts Institute of Technology.
What is Brightpick? What types of market applications or specific warehouse workflows is it designed for?
Brightpick is a spinoff from Photoneo. The solution leverages the 3D perception and robotic picking technology that is the core of Photoneo. It’s a warehouse automation solution specifically designed for order fulfillment and targeting different verticals like e-grocery, third-party logistics, and e-commerce.
The Brightpick solution is optimized to handle smaller objects like small electronics, grocery items, and similar items that can be stored in bulk in inventory totes.
Describe the typical fulfillment workflow for a Brightpick autonomous mobile robot (AMR).
The Brightpick AMR travels through the warehouse as it builds one discrete order similar to a human picker. The warehouse inventory needs to be organized into a set of totes and the individual items have to be “toteable.”
Leveraging an onboard mobile manipulator, the eaches picking is done directly from the bulk inventory totes pulled from the shelf while the BrightPick AMR is stationary in the warehouse aisle.
What typical size of totes can Brightpick handle?
We like to have one uniform-size tote for the warehouse. The preferred totes are usually 60 by 40 cm [23.6 by 15.7 in.], and the height of the tote could vary, depending on the items stored. The totes can be split into multiple compartments. The storage requirements depend on the order frequency of different items. Space utilization is key, and having compartmentalized totes helps to improve the efficiency of the solution.
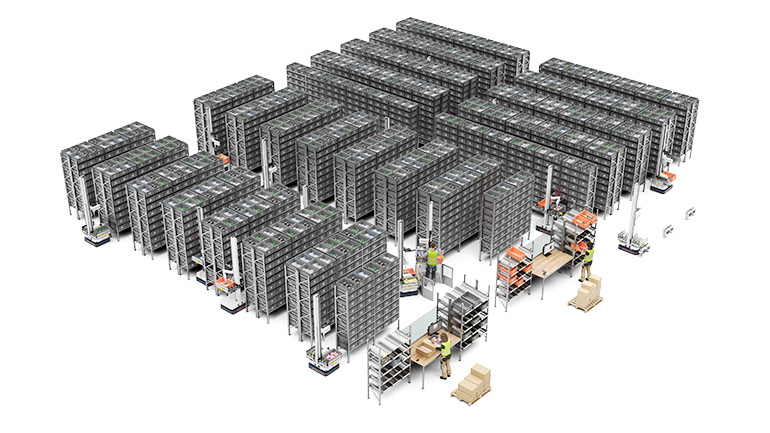
Brightpick picker AMRs traverse the warehouse to pull individual items for customer orders. | Credit: Brightpick
What was the design process and the “Aha” moment for the Brightpick solution? Photoneo’s strength is in 3D vision, 3D perception, and robot vision guidance, especially for applications like bin picking.
Over the years, we have been studying how fundamentally difficult fulfillment is, and we decided it’s a big enough problem to be solved. We made this robot from the ground up utilizing all the Photoneo technologies into Brightpick.
We already had all of the necessary capabilities to accomplish this difficult task. Starting with our 3D cameras, over time, we developed our machine learning engine.
In grocery, we can pick around 80% of items that we see from the tote. We like to call it “fully automated fulfillment,” which is not completely true, but our definition of fully automated fulfillment is in principle that we can automate 95% of the entire process.
What are some of the issues that you’ve had to solve for this new automated workflow for fulfillment?
Orchestrating the robot is a difficult problem. We use specific algorithms in the classical “traveling salesman problem” to send the individual robots out for each SKU-picking task. If it would be just one robot, it would be simple.
One of the most complex problems we are solving is something we call time-space planning. When you have a fleet of robots, each robot could just solve a traveling salesman problem individually, but here we have to orchestrate the robots as a fleet.
One issue we have is that the robots might be competing for a given aisle, or even a given SKU, and we have to avoid that. So this is a super complex problem. As the robots travel through the warehouse, we have to plan their paths, plan the picking dwell times, and negotiate conflicts where two robots want to be in the same place at the same time.
Learn from Agility Robotics, Amazon, Disney, Teradyne and many more.
There are some other robots on the market, like InVia’s AMRs, which pull inventory totes from a shelf and transport them to an item-picking location, then return the inventory to the warehouse shelf. Is this similar to an automated storage and retrieval system (ASRS)? Do you categorize Brightpick as an ASRS-type system?
I might categorize the InVia solution as a goods-to-person [G2P]-type solution, like Kiva Systems. The other emerging category is “robots to goods,” and maybe that’s the right category for Brightpick.
With the Kiva-like solutions, the robots have to move bulk items long distances just to pick a single SKU for a customer order. But the most important part is that the robot has to bring this tote back to its long-term storage location in the warehouse. So bulk items are continuously moved to the picker, back to the inventory location, and back to the picker, and so forth.
So we believe that the BrightPick solution fundamentally and mathematically requires 40% to 50% or less travel. And that means that you could have fewer robots deployed on the floor. Our goal is to create a super-efficient system. We have to achieve these efficiencies.
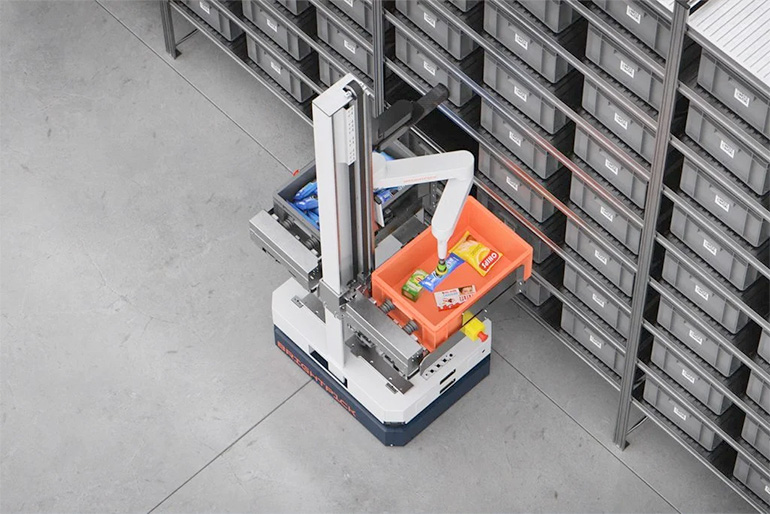
The Brightpick Autopicker AMR pulls bulk item totes from the warehouse shelf to singulate individual customer items. | Credit: Brightpick
Brightpick uses a SCARA manipulator to pick and place individual items from totes. Why a SCARA robot versus a six-axis manipulator?
Maybe this isn’t the interesting answer, but it comes down to cost. We made a trade-off between the complexity of AI and algorithms and the cost of the manipulator. The choice to use a SCARA manipulator for picking means that the AI, gripper, and algorithms behind it are more complex for the bin-picking operation.
It took us a few years, and we designed around 200 grippers with different variations and different tricks. What we ended up with is something that is a good balance between complexity, cost, and capabilities.
Today, we cannot be 100% confident of picking every item, but then no solution can. I think humans can still serve as a fallback solution by engaging a nearby associate to help pick an item. I would say for customers, end-to-end reliability is much more important than the fact that you can pick like 99.9% of the items.
Do you use a single gripper for all the items, or are you doing a tool change for different kinds of parts?
We have we have both concepts. We do a lot of different gripper concepts including multiple suction cups, gripper exchangers, and suction-cup exchangers.
So all of this is possible. It looks like not every warehouse requires such flexibility. Ultimately, we will end up with something like a gripper exchanger with two or three fingers.
So you defined this new segment a few minutes ago as a “robot-to-goods” solution. What makes this type of system viable now?
The robot you see here is probably the fifth generation. It’s not designed to do 50 different things; it’s not like a general-purpose humanoid. On the other hand, what we have done is optimize the cost of each element of the robot to reduce the cost of the required functionality.
I’m super proud of what our team was able to do on the hardware design side and with procurement.
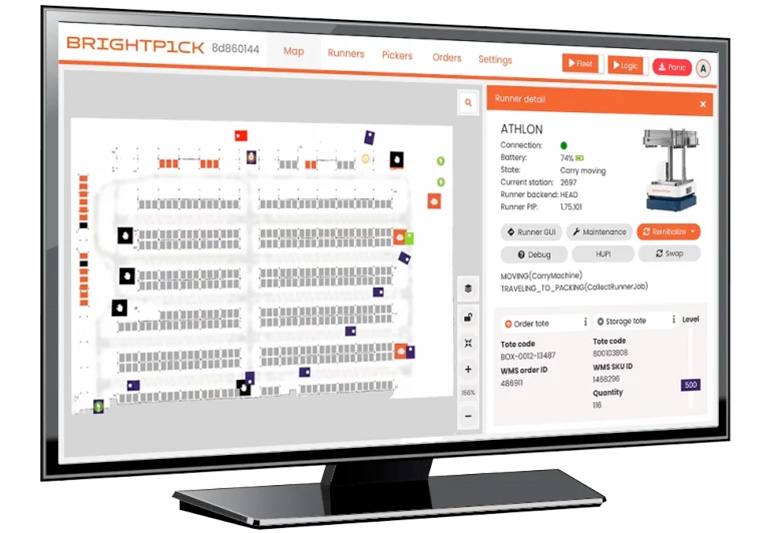
Brightpick includes software and optimization algorithms to control material flow and the operation of all of the individual robots on the warehouse floor. | Credit: Brightpick
Bin picking with mobile manipulation has been a “holy grail” problem. Is there a breakthrough in this design that you’re particularly proud of?
It comes down to a holistic view of all of these latest developments in artificial intelligence. You have to have the right gripper, the right path planning, the right AI, and the right concept. Everything has to be perfect. And if one part is missing, suddenly, it is not feasible.
On the AI side, this was not possible five to six years ago. The serious breakthroughs enable machine learning to generalize to unseen items. I think that this is so powerful. This is just the beginning.
For example, IAM Robotics struggled for years to make mobile manipulation viable. Why do you think its approach wasn’t successful?
I think it’s probably two things. First, if you buy a six-axis robot from a major supplier, you can get a very good product. But the price is prohibitive. I think there’s simply no way that you can make it happen today.
Second, IAM Robotics was picking directly from shelves. This is complex a very costly replenishment process. Our replenishment solution is to just dump it into the tote.
Replenishment in our system is probably like five to six times more efficient because robots will slot a given tote into the right place in the warehouse. We are trying to analyze the frequency at which items are picked to organize where items should go on the shelf.
You’ve built an algorithmic fulfillment heatmap of common items. Are you constantly reorganizing the warehouse to make it more efficient for the robots to pull items?
Yes, exactly. In classical G2P systems, you need to minimize the distance you need to travel with the robots. So moving high-frequency SKUs closer to the human pickers is very obvious here.
With these older G2P robots, they are all equal, standalone, and self-sufficient. All the robots can do all the processes. They have their trajectories.
It’s not so obvious now to ask: “Do I want to create a hotspot somewhere?” Probably not, because I can steer too many robots to the same aisle or same spot, and this congestion creates a new problem.
You have to somehow balance in a big warehouse, between shortening distances and spreading those items out too far. It’s a key optimization problem.
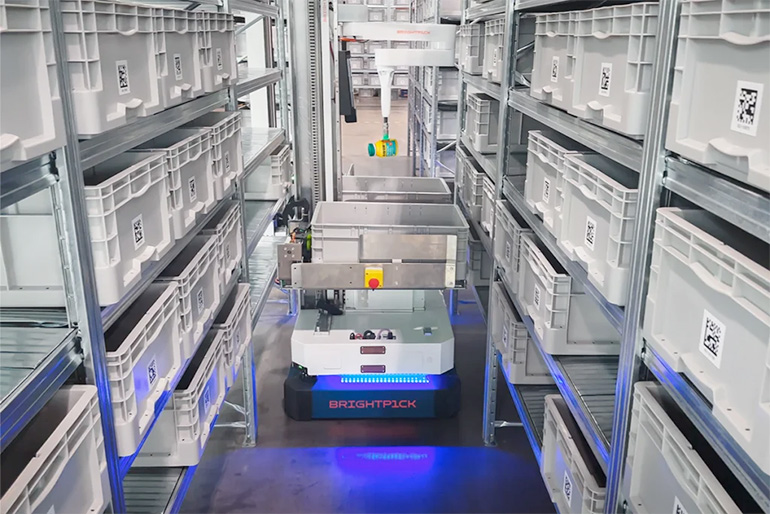
The Brightpick Autopicker is designed for narrow aisles. | Credit: Brightpick
Do you leave space in the aisles for two-way traffic to avoid potential collisions or path conflicts?
Two years ago, most of the aisles were one-way. Today, almost all of them are two-way. With strong planning algorithms, we can decide the paths for the robots to avoid potential conflicts in the aisles.
We try only to have one robot in each aisle at a time. But this time-space planning problem is a hard problem to solve. There are multiple levels to it. But safety with robots and collaborative manipulators is critical.
Can you share some of your early customer deployments?
Our biggest customer today is Rohlik Group, one of the fastest-growing e-grocers in Europe. These are big orders and mostly ambient items. We are deploying a chilled segment in the upcoming weeks.
Grocery is very interesting. If you imagine your family buying 100 items per week, that’s a lot of picks per order. If you compare that to other e-commerce, it could be that groceries are bigger than the entire e-commerce segment. I think groceries are one of our top targets.
We are finishing a new deployment for a pharma client. In this use case, it’s a lot of smaller boxes and a high number of SKUs. When we were designing the Brightpick system, this was something that we had in mind.
When looking at an application, we look at the number of picks per line. For example, if you ask me for an application having 100 items per line, that means a lot of picks for the given SKU.
Yes, that’s probably not optimal, and no human is much faster. We’ve been counting 100 items, you will still do it five times quicker than the robot. We are always trying to find the best match in order profile.
Listen to the interview in its entirety here:
Tell Us What You Think!