The autonomous vehicle and robotics sectors often employ LiDAR as the primary system navigation sensor. But cameras and vision-based perception will increasingly serve as the technological underpinning for mobile robots going forward.
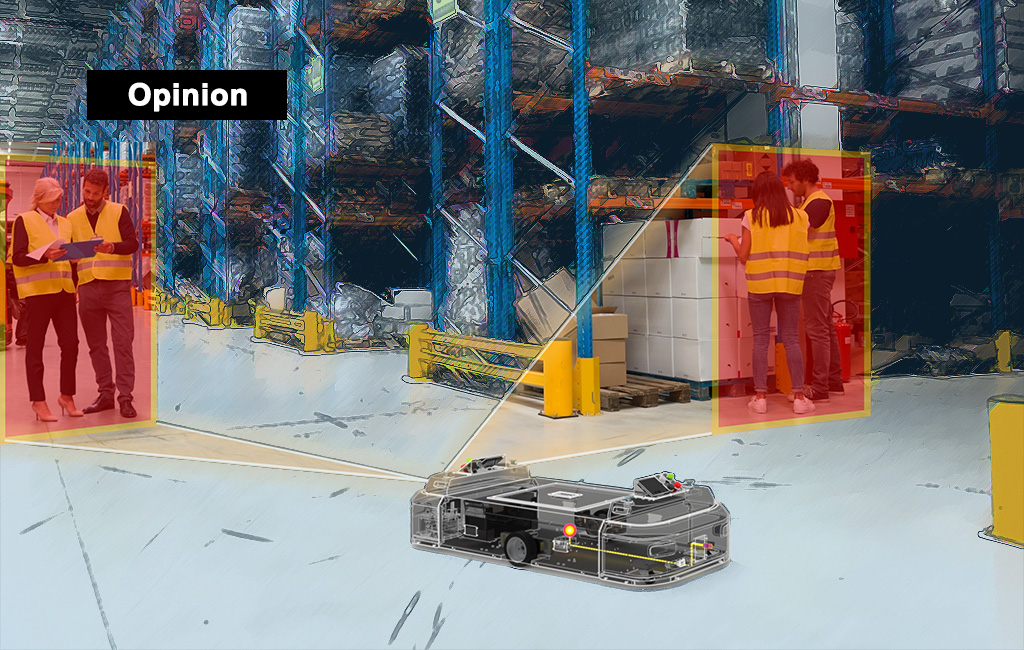
Listen to this article
|
Most autonomous vehicle manufacturers incorporate high-end 3D LiDARs, along with additional sensors, into their vehicles so that they are provided with enough data to fully understand their surroundings and operate safely. Yet in April 2019, Elon Musk famously told attendees at Tesla’s Autonomy Day that LiDAR is a “fool’s errand”—and that anyone relying on it is “doomed,” referring to Tesla’s preference for vision-based perception.
The LiDAR / vision debate continues to this day. But since that time there has been a steadily increasing emphasis on cameras and computer vision in the autonomous vehicle market.
Vision-based Navigation for AMRs
Recently, the same debate has emerged in the mobile robot market where traditional 2D LiDARs have been the prevailing navigation sensor for decades. Some AMR manufacturers, including Canvas Technology (acquired by Amazon), Gideon Brothers, and Seegrid, have already developed AMRs with varying degrees of vision-based navigation.
One reason why these AMR companies have opted for camera-based navigation solutions is the lower cost of vision systems compared to LiDAR. But the most compelling reason is the ability of vision-based systems to enable full 3D localization and perception.
Seeking Alternatives
3D LiDAR is also an option for robotics developers looking to add 3D perception capabilities into their systems. But while the price of 3D LiDAR solutions has dropped over the past few years, the total system cost for 3D perception continues to be many thousands of dollars.
For the robotics sector, the cost of automotive grade 3D LiDAR is usually prohibitive. As a result, robot manufacturers continue to seek less expensive alternatives to 3D LiDAR for 3D perception.
Cameras can see natural features on the ceiling, floor, and far into the distance on the other side of a facility.
Camera-based Vision Systems
Camera-based vision systems are inherently up to the perception challenge since they can ‘see’ and digitize everything in their field of view. Leveraging economies-of-scale from other industries, even cameras costing under $20 provide enough resolution and field-of-view to support robust localization, obstacle detection, and higher levels of perception.
Localization in Challenging Environments
Another important advantage of vision-based navigation is the ability to handle challenging environments where LiDARs lose robustness. The classic example is a logistics warehouse where rows of racks and shelving systems are repeated throughout the facility.
Cameras can also see natural features on the ceiling, floor, and far into the distance on the other side of a facility. But the 2D ‘slice’ of the world that a LiDAR can see is simply not enough to distinguish between the different, repetitive features in these environments. As a result, LiDAR based robots can get confused or even completely lost in many situations.
These same challenges also apply to open or highly dynamic environments like cross-docking and open warehousing facilities. The ‘slice’ that LiDAR saw and interpreted during their last visit may now be open space – or something else altogether.
Ultimately, to achieve truly intelligent autonomous behavior, navigation systems must deliver human-level, 3D perception.
3D Perception and Scene Understanding
Finally, and most importantly, vision-based perception can enable capabilities that other types of sensors are fundamentally incapable of. Ultimately, to achieve truly intelligent autonomous behavior, navigation systems must deliver human-level, 3D perception. For example, since they can detect texture and color, cameras are able to distinguish between the edge of a sidewalk and the edge of the road. This can create significant safety advantages for delivery robots because the robot can use this visual information to precisely navigate along its edge, just the way a human would.
This capability is useful in warehouses and manufacturing facilities where pedestrian paths are defined with lines and floor markers. Camera-based systems can even read signs and symbols that can alert both humans and robots to temporary closures, wet floors, and detours. Vision-based navigation systems are also able to work in both indoor and outdoor environments – opening up new use cases and applications.
The Challenge
Converting the large volume of data from cameras into 3D perception on low-cost hardware is a monumental technology and engineering challenge. The process requires a significant AI, computer vision, and sensor fusion expertise on the part of engineers, along with the availability of enabling technologies.
Thankfully, robust, performative solutions for camera-based 3D perception is now to robotics engineers. For example, RGo Robotics’ solution, Perception Engine, is a full-stack software solution that enables manufacturers to deliver next-generation capabilities rapidly. It is able to utilize just a single camera in some applications to achieve precise 3D localization and perception. Its wide field of view camera is also able to recognize humans and other obstacles around it. This level of scene understanding allows mobile robots to behave more naturally and collaboratively around humans.
Additional Modalities
All said, there remains significant value in traditional sensor modalities including LiDAR. Recent advancements in low-cost MEMS 3D LiDARs is encouraging and, when combined with cameras, could add cost effective robustness and rich 3D mapping capabilities to robotics systems.
But Musk was correct in saying that cameras and computer vision should serve as the foundation of any mobile robot navigation system. The next few years will certainly see dynamic changes as the state-of-the-art evolves with advances in both the autonomous vechicle and robotics industries.
About the Author
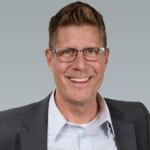
As SVP Marketing & Business Development, Peter Secor is responsible for building RGo Robotics’ brand and identifying new customer and market opportunities for the company. Prior to RGo, he held transformative positions with companies at the leading edge and intersection of IoT, industrial automation, robotics and 3D printing including iRobot and Stratasys. Secor started his career as a management consultant where he specialized in corporate strategy development and M&A for Fortune 500 companies in the industrial automation market including Rockwell Automation, Siemens and Honeywell. He holds a BS in Mechanical Engineering from the University of New Hampshire and an MBA from Columbia University’s Columbia Business School with a concentration in technology growth marketing.